일 | 월 | 화 | 수 | 목 | 금 | 토 |
---|---|---|---|---|---|---|
1 | ||||||
2 | 3 | 4 | 5 | 6 | 7 | 8 |
9 | 10 | 11 | 12 | 13 | 14 | 15 |
16 | 17 | 18 | 19 | 20 | 21 | 22 |
23 | 24 | 25 | 26 | 27 | 28 |
Tags
- cellranger
- 비타민 C
- EdgeR
- Batch effect
- single cell
- single cell analysis
- ngs
- ChIPseq
- julia
- python matplotlib
- scRNAseq analysis
- drug muggers
- HTML
- pandas
- CSS
- js
- github
- CUTandRUN
- MACS2
- drug development
- javascript
- matplotlib
- single cell rnaseq
- PYTHON
- Bioinformatics
- DataFrame
- CUT&RUN
- Git
- 싱글셀 분석
- scRNAseq
Archives
- Today
- Total
바이오 대표
[ Text Similarity ] String-Based Similarity (Cosine, Jaccard) 본문
Master dissertion
[ Text Similarity ] String-Based Similarity (Cosine, Jaccard)
바이오 대표 2022. 1. 11. 01:02
Text Similarity 를 구하는 방법에는 String-based, Corpus(말뭉치)-based, Knowledge based가 있다
논문에서 Side Effect to Side Effect, Disease to Disease Similarity 를 위해 간단히 String-Based Text Similarity 를 적용하였다.
String-Based Text Similarity:
[1] Character-based
[2] Term-based ( Cosine Similarity, Jaccard Similarity)
Jaccard Similarity | number of shared terms over the number of all unique terms in both strings |
Cosine Similarity | similarity b/w two vectors of an inner product space that measures the cos of the angle between them |
해당 방법은 각도 기반 유사도 측정법이다. 유사도 측정법에는 크게 거리기반 그리고 각도 기반 측정법이 있다.
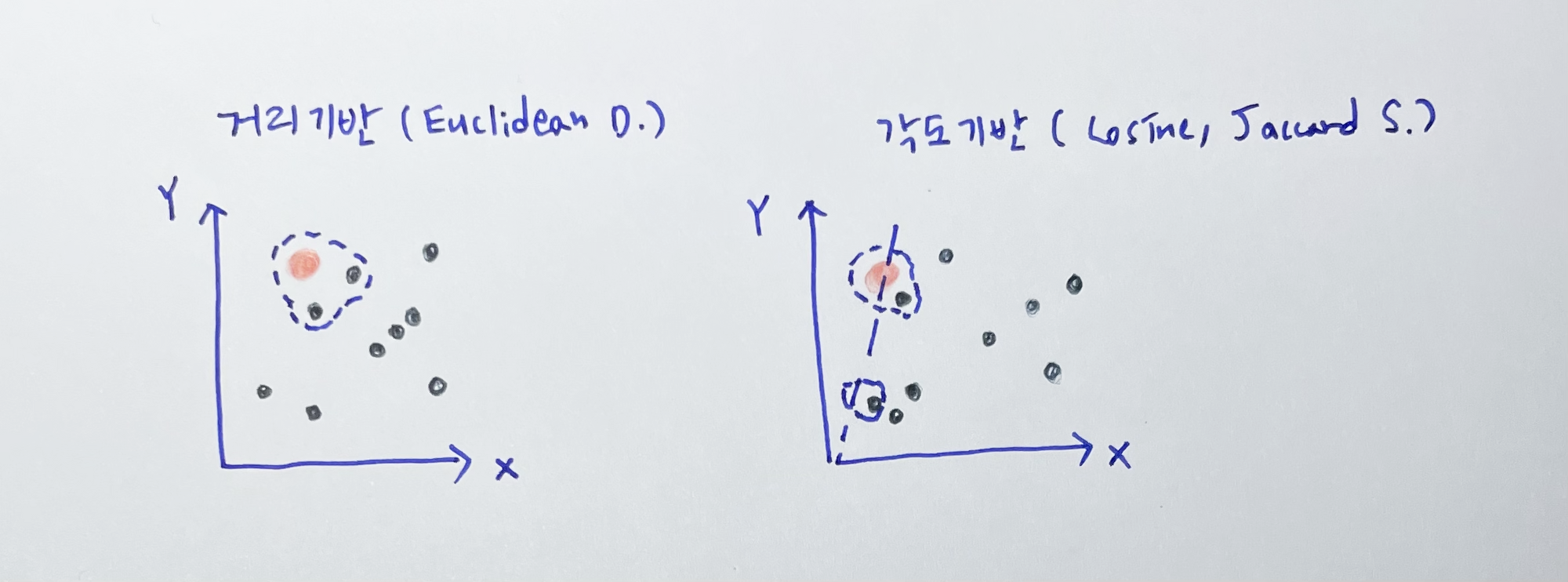
[1] Jaccard Similarity
def Jaccard_Similarity(doc1, doc2):
# List the unique words in a document
words_doc1, words_doc2 = set(doc1.lower().split()), set(doc2.lower().split())
# Find the intersection of words list of doc1 & doc2
intersection = words_doc1.intersection(words_doc2)
# Find the union of words list of doc1 & doc2
union = words_doc1.union(words_doc2)
# Calculate Jaccard similarity score using length of intersection set divided by length of union set
return float(len(intersection)) / len(union)
doc_1 = "Data is the new oil of the digital economy"
doc_2 = "Data is a new oil"
Jaccard_Similarity(doc_1,doc_2) # 0.44444
[2] Cosine similarity
from numpy import dot
from numpy.linalg import norm
def cos_sim(A, B):
return dot(A, B) / (norm(A) * norm(B))
A = [0.123, 0.456, 0.789]
B = [0.345, 0.765, 0.987]
result = cos_sim(A,B) # result = 0.9821175
import sklearn.metrics.pairwise
result2 = sklearn.metrics.pairwise.cosine_similarity([A,B])
result2 # array([[1. , 0.98211752],
# [0.98211752, 1. ]])
다양한 Similarity 방법들을 아래 논문에서 확인 할 수 있다.
Reference
[1] Gomaa, W.H., & Fahmy, A.A. (2013). A Survey of Text Similarity Approaches. International Journal of Computer Applications, 68, 13-18.