일 | 월 | 화 | 수 | 목 | 금 | 토 |
---|---|---|---|---|---|---|
1 | 2 | 3 | 4 | |||
5 | 6 | 7 | 8 | 9 | 10 | 11 |
12 | 13 | 14 | 15 | 16 | 17 | 18 |
19 | 20 | 21 | 22 | 23 | 24 | 25 |
26 | 27 | 28 | 29 | 30 | 31 |
- single cell rnaseq
- scRNAseq
- Bioinformatics
- javascript
- EdgeR
- MACS2
- julia
- single cell
- matplotlib
- CUT&RUN
- single cell analysis
- scRNAseq analysis
- 싱글셀 분석
- js
- python matplotlib
- DataFrame
- drug development
- github
- cellranger
- Git
- drug muggers
- PYTHON
- pandas
- CSS
- HTML
- Batch effect
- ChIPseq
- ngs
- CUTandRUN
- 비타민 C
- Today
- Total
목록Mathematical Optimization (11)
바이오 대표
! Automatic differentiation 을 이용해서 ML 과, optimization 에서 활용할 수 있다. physical engines to learn robot controls differentiating protein structures modeling of molecular mechanisms
Machine learning 중 Neural Network 를 이용한 트레이닝에서 backpropagation 을 이용해 모델의 parameters 을 재조정한다. Backpropagation이 reverse differentiation 이다. julia> using Zygote julia> f'(a) julia> Zygote.gradient(f, a) julia> Zygote.gradient((x1, x2) -> (x1^2 + x2^2 - 0.1x1*x2) / (x1 + 1.0), ⋅ 0.2, 0.3)
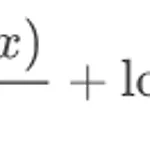
Computer algebra systems 을 이용해서 굳이 머리쓰지않고 쉽게 미분을 할 수 있다. Maple Sympy (Python) Mathematica Maxima Symbolics (Julia) julia> using Symbolics julia> f(x) = log(x) + sin(x) / x julia> Dx(f(x)) julia> df_sym = expand_derivatives(Dx(f(x))) julia> df = build_function(df_sym, x) |> eval #builds an expression and turns it into a function julia> df(2.0) # 0.06460222502000837
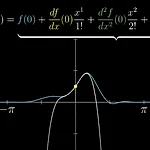
몇년 동안 대학, 대학원 공부를 하면서 참 많이도 다뤘던 taylor series 이지만 제대로 개념을 챙긴적이 없다. 그러다가 교수님이 알려주신 동영상,,,, 갓 유투브 사랑합니다. approximate function 만들기 - 확실한 point, 에를 들어 cos graph = 1 at x = 0 - consider tangent line (derivative) - consider positive/ negative rate or slope (second derivative) 더 나아가보면 요소를 추가하면 추가할수록 해당 그래프와 더 비슷한 approximate function 을 만들 수 있고 이를 Taylor polynomical 이라 칭한다 => convergence to answer value..