일 | 월 | 화 | 수 | 목 | 금 | 토 |
---|---|---|---|---|---|---|
1 | ||||||
2 | 3 | 4 | 5 | 6 | 7 | 8 |
9 | 10 | 11 | 12 | 13 | 14 | 15 |
16 | 17 | 18 | 19 | 20 | 21 | 22 |
23 | 24 | 25 | 26 | 27 | 28 |
- single cell rnaseq
- ngs
- drug development
- Batch effect
- CSS
- matplotlib
- CUTandRUN
- drug muggers
- github
- 싱글셀 분석
- MACS2
- DataFrame
- cellranger
- EdgeR
- Bioinformatics
- js
- Git
- julia
- python matplotlib
- scRNAseq analysis
- single cell analysis
- ChIPseq
- 비타민 C
- javascript
- CUT&RUN
- HTML
- pandas
- scRNAseq
- PYTHON
- single cell
- Today
- Total
목록분류 전체보기 (216)
바이오 대표
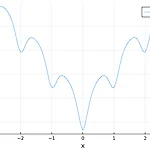
Ackley function은 minimum, 최적화, 를 찾기위한 테스팅을 위해 만들어내는 local minima 가 많은 그래프이다. julia> using Plots, PlutoUI julia> begin function ackley(x; a=20, b=0.2, c=2π) d = length(x) return (-a * exp(-b*sqrt(sum(x.^2)/d)) - exp(sum(cos.(c.*x))/d)) end ackley(x...; kwargs...) = ackley(x; kwargs...) end ackley (generic function with 2 methods) julia> ackley(1.0) 3.6171031099813176 julia> plot(ackley, -pi, pi, ..
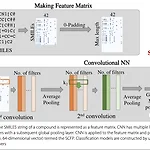
다양한 fingerprint 와 graph convooution architecture을 이용한 DL 모델 발전. 하지만 이는 (1)분자 비대칭성(chirality of compounds), (2) effective feature을 발견하느냐에 따라 효과적일수도있고 아닐수도 있다. Model: CNN (input = SMILEs notation) Goal: Compounds Classification (Chemical Motif detection with the learned feature) dataset: TOX 21 dataset SMILES 장점: Linearly represents 로써 low dimensional representation 이다...
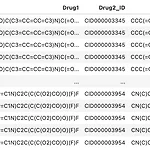
Drug Data 만지다가,,, 괜히 리스트 만들어서 병합하고 버리고 하다가 26시간 걸린거 pandas 및 dataframe 으로 건드니까 5분으로 해결된거에 화가나서 같은 실수를 반복하지말자며 끄적끄적 ,,, DL 은 장비빨,,, 장비가 부족하면 Complexity 를 최대한 줄이자 df.Drug1.unique( ) df.duplicated( ) df.duplicated(subset=['Drug1', 'Drug2']) df.drop(["Drug1_ID", "Drug2_ID"]) df.drop_duplicates(subset=['Drug1', 'Drug2']) pd.merge( df1, df2, on=["Drug1", "Dr..
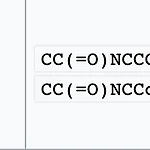
## DB 데이터베이스와 ML 을 이용한 target 에 적합한 chemical compound (drugs) 찾기 - CheMBL *chemical "SMILES" : simplied molecular-input line entry system (molecule들의 구조 structure 을 컴퓨터가 인식하기 쉽고 문자열로 나타낸 것) - Descriptors 이용 가능 ex) Lipinski descriptors 를 이용해서 먹는 약 (orally active drug)가 될 자격이 있는지 확인 가능 Lipinski descriptors 포..