일 | 월 | 화 | 수 | 목 | 금 | 토 |
---|---|---|---|---|---|---|
1 | 2 | 3 | 4 | 5 | ||
6 | 7 | 8 | 9 | 10 | 11 | 12 |
13 | 14 | 15 | 16 | 17 | 18 | 19 |
20 | 21 | 22 | 23 | 24 | 25 | 26 |
27 | 28 | 29 | 30 |
- github
- CUTandRUN
- CUT&RUN
- 싱글셀 분석
- javascript
- Bioinformatics
- PYTHON
- scRNAseq
- matplotlib
- Batch effect
- drug development
- pandas
- ngs
- drug muggers
- scRNAseq analysis
- Git
- single cell
- CSS
- DataFrame
- cellranger
- MACS2
- julia
- js
- 비타민 C
- EdgeR
- HTML
- python matplotlib
- single cell rnaseq
- ChIPseq
- single cell analysis
- Today
- Total
목록전체 글 (217)
바이오 대표
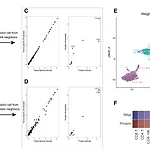
Introduction Mutimodals 을 이용한 싱글셀 분석. 해당 논문에서는 “weighted-nearest neighbor(WNN)” 을 이용하여 integrate multiple data types (joint definition of cellular states)를 구한다. WNN 은 각 cell-specific modality weights를 구하는 unsupervised learning 이다. Results 대부분 CITE-seq을 기준으로 진행하였다. CITE-seq을 이용하면 scRNAseq 데이터와 세포 표면에 붙어있는 protein들의 정보를 알 수 있다. 여러 modality를 이용했을때, 각 세포를 더 잘 reflect 할 수 있는 modality 에 더 큰 weight 부여해..
Abstract 10x Genomics (droplet-based system) 3’ mRNA counting of tens of thousands of single-cell per sample 8 samples at a time 50% cell capture efficiency ~ 250k single cells across 29 samples 로 [1] sensitivity, [2] ability to detect rare population를 파악하고, 68k PBMC cells을 이용하여 [3] ability to characterize large (immune) population 를 실험하였다. 또한 골수 이식환자 데이터의 sequence variation을 이용하여 [4] host/donor..
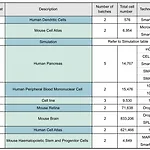
Abstract 해당 논문에서는 현존하는 14개의 batch correction 방법들을 비교분석한 결과를 다음과 같은 3개의 카테고리에서 보여준다. [1] computational runtime, [2] 큰 데이터셋을 다루는 능력치 [3] 순수 cell type 을 잘 보존하면서 batch correction 을 하는지. 결론적으로는 Harmony, LIGER, Seurat 3 을 추천한다. Introduction 현재 single cell 데이터 batch correction 에 주로 사용되는 알고리즘들을 소개한다. MNNs (Haghverdi et al.) 합치고 싶은 2개의 데이터에서 mutual nearest neighbors (MNNs) 를 계산한다. 그리고 해당 MNNs (list of pa..
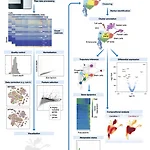
해당 글은 "Current best practices in single-cell RNA-seqanalysis: a tutorial" https://www.embopress.org/doi/epdf/10.15252/msb.20188746" 를 요약 정리한 글입니다. 전체 흐름 scRNAseq analysis tools increase → lack of standardization (+ dependency of language) The paper introduces the typical scRNAseq analysis steps as current best-practice recommendations. 처음 분석을 하시는 분들께 어느정도 가이드라인이 되는 페이퍼라고 생각한다. count matrix pre-p..
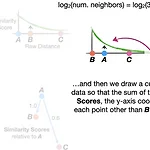
- PCA - 해당 글에서는 UMAP 관련해서 좀 자세히 다뤄보려 한다. 다음 내용은 StatQuest 을 참조하였다. UMAP 을 이용해서 High dimension 데이터를 low-dimension에 표현할 수 있다. How? 전체적인 그림은, 낮은차원에서 point를 움직여서, high dimention 에서와 비슷한 모습을 보이도록 조정하는 방법이고 이를 Similarity score 을 계산하여 사용한다. 1. High-dimention points 에서 서로간의 distance 를 계산한다. 2. High-dimention neighbor 숫자 (default 15) 에 따라 log2 (#of neighbor) 을 이용하여 curve 를 그리고, 각 포인트의 similarity score 을 ..
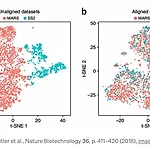
해당 글은 지마님의 블로그의 Batch effect https://ruins880.tistory.com/88 를 참고하였습니다. 좀 더 자세한 사항은 지마님의 블로그를 참고바랍니다. Normalization vs Batch effect correction in scRNAseq Normalization: 라이브러리 제작, dropout, 유전자 길이, GC 비율 등에 따른 증푹 치우침 (amplification bias) - raw count matrix 이용 Batch Effect correction: 실험 설계 및 실험 과정 (시퀀싱 기계, 실험 시각, 시약, 실험실) 에서 오는 변이 통제 - 차원이 축소된 데이터 이용 (계산 시간 줄이기 위하여) ⇒ batch effect 가 제거된 결과는 차원이 축..